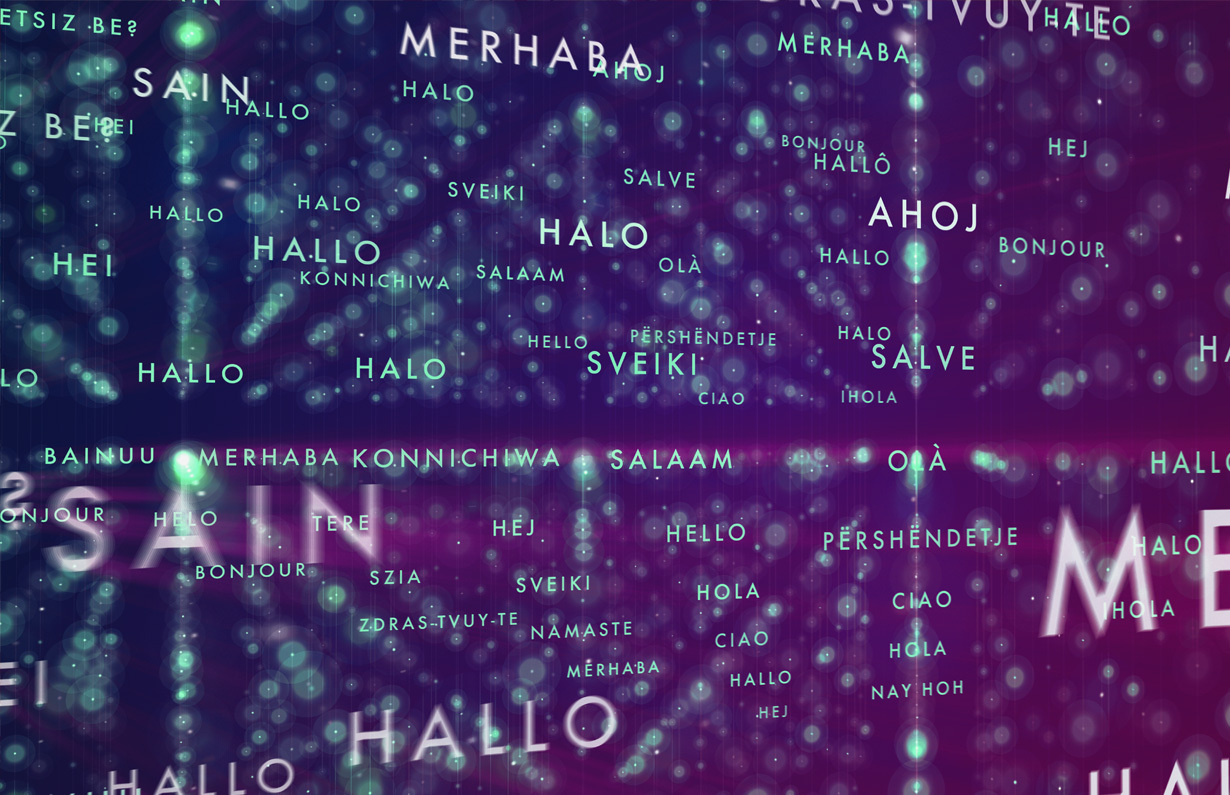
Machine translation – the future is already here (and humans are still at the heart of it)
The use of machine translation is becoming more and more common in every area – from business to entertainment. But can the results meet the highest quality standards?
The translation and language services market has been growing steadily for years now, with certain sectors in particular enjoying substantial growth since the start of the pandemic in 2019, most notably healthcare and social media. While other sectors have been hit by the spread of Covid (e.g. travel, events and transport), overall the translation and related services industry now turns over tens of billions of dollars every year, proving that it is in excellent health.
That growth is driven by a number of factors, with three key dynamics emerging:
- globalization, and the accompanying need to make content linked to business, digital services, culture and leisure accessible to many more communities;
- the development of information technologies – web-based and beyond – such as augmented reality or artificial intelligence, which are increasingly being used in consumer products and services (from online banking to in-car devices and social media feeds);
- the new frontiers being explored by computational linguistics, underpinning the most powerful machine translation (MT) methodologies.
From CAT tools to machine translation
To meet the huge demand for 'content localization' and their accompanying delivery times, professional translators and LSPs have gradually adopted new workflows and technology over time. CAT tools (Computer-Assisted Translation) – both software- and cloud-based – have made it possible to cut delivery times and optimize the working process by leveraging translation memories and termbases, as well as other functions.
In more recent times, CAT tools have been supplemented by programs – usually SaaS-based – which have gone a step further by enabling a computer to complete the entire translation itself. Google Translate and DeepL are two of the best known. While until recently the consensus was that the results still leave something to be desired, some studies are now showing a widespread level of satisfaction among professional users, who judge many machine translations in a range from 'acceptable' to 'very good'.
When you then add in human skills to supplement the MT, transcending the age-old conflict between human and machine translation by applying a sensible mix of approaches, the opportunities for those capable of exploiting this new scenario also become 'very good', potentially spanning every kind of business. Cutting the time and cost of your translations not only increases your profits but allows you to open up to even more global audiences and markets, turning them into new opportunities for growth and profit.
The evolution of machine translation
In reality, the origin of machine translation is anything but recent, since the first experiments date back to the 1940s. Despite the less than encouraging results, research into the subject continued steadily and resurfaced with fresh vigor at the turn of the century with new models. Three main types of MT have emerged since the 1960s:
- rule-based machine translation;
- statistical machine translation
- neural machine translation.
The first method (rule-based) uses a set of syntactic and lexical rules to break down the sentence, identify each word's function and reconstruct the proposition in the target language.
Statistical MT, popular since the start of the new millennium, is essentially based on huge corpora of translated texts, which the computer uses to determine the best choice based on mathematical analysis of occurrences and contexts.
The newest – and most promising in terms of quality of results – is neural machine translation, which is based on concept hierarchies. The machine can perform complex functions (such as translation) because they are described in a formal language based on hierarchical dependencies, which also allow for continuous remodeling and ever deeper nested concepts, enabling the artificial intelligence to learn continuously (Deep Learning). Here is a detailed – and highly technical – article published by ScienceDirect on the mechanics of linguistic automation..
Neural MT forms the basis for the proliferation of online translation services. But is also the foundation, for example, of the new glasses that Google announced this year allowing two or more people speaking different languages to talk to one another, or of Apple's promised service providing simultaneous subtitles for any content on its main devices. Not to mention the universe of nascent possibilities heralded by the metaverse, where the language barrier is indeed among the frontiers that Zuckerberg's Meta aims to transcend.
So, is MT a friend or a foe?
As we said, the best thing is to move beyond a binary position and instead think of an ecosystem in which people and machines are parts of a whole mechanism designed to deliver the optimal result.
Ultimately, machine translation today presents a significant opportunity for business and professionals, who are not replaced by it but can use it as a tool – a way to make better decisions, optimize time and resources and, in the end, produce more effective content. And content increasingly goes beyond text: graphics, audio, video, code, metadata - all areas in which these new forms of content generation are yielding very interesting results.
Even so, it is always a 'human-in-the-loop approach that makes artificial intelligence an even more formidable tool. Furthermore, growth forecasts for the language and translation services market are more than encouraging. According to this analysis by Nimdzi in 2022 the industry will be worth 64.7 billion dollars and is projected to reach 84.9 billion in 2026 at an average growth rate of 7% (!). It is therefore better to join forces with the machines rather than fight them - if anyone still considers fighting them an option.